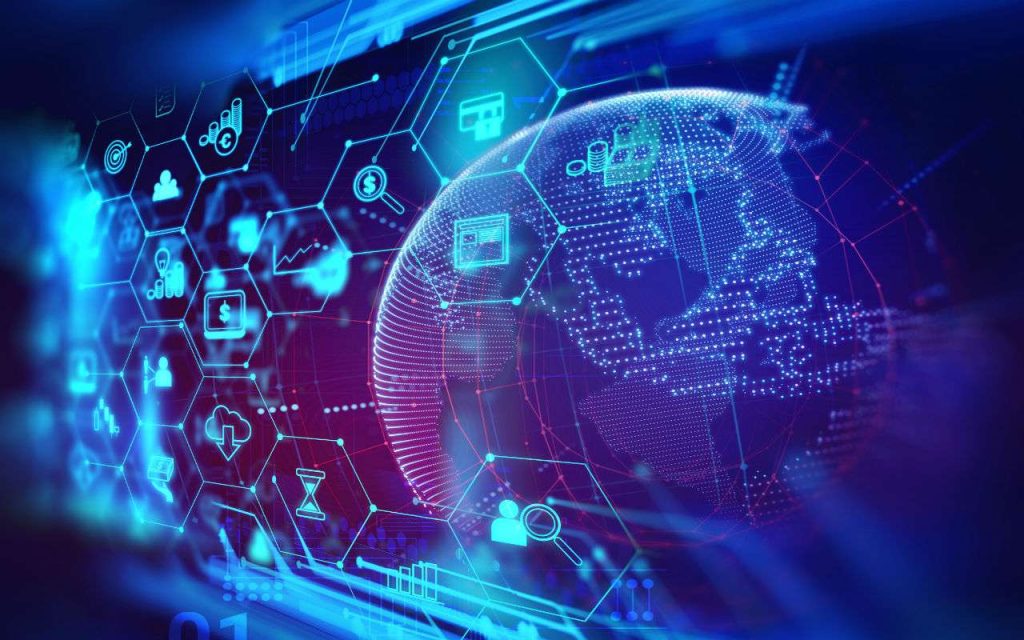
In the advancing world of technology where LLMs are being incorporated into every aspect of our daily lives and tech giants like Nvidia and OpenAI are engulfed in the frenzy of Artificial General Intelligence (AGI), there is a high tendency of the average internet user coming across the term “Data Science”.
What is Data Science?
Data Science is an inter-disciplinary field of mathematics and statistics that employs the use algorithms built on these fields to explore, clean and extract profitable insights from a collection of data. The insights gotten from this data can be used to create new business strategies, make business decisions or train machine learning models to perform certain tasks.
It is the domain of study that deals with vast volumes of data using modern tools and techniques, including essential data sciences skills, to find unseen patterns, derive meaningful information, and make business decisions.
– Simplilearn
Who is a Data Scientist?
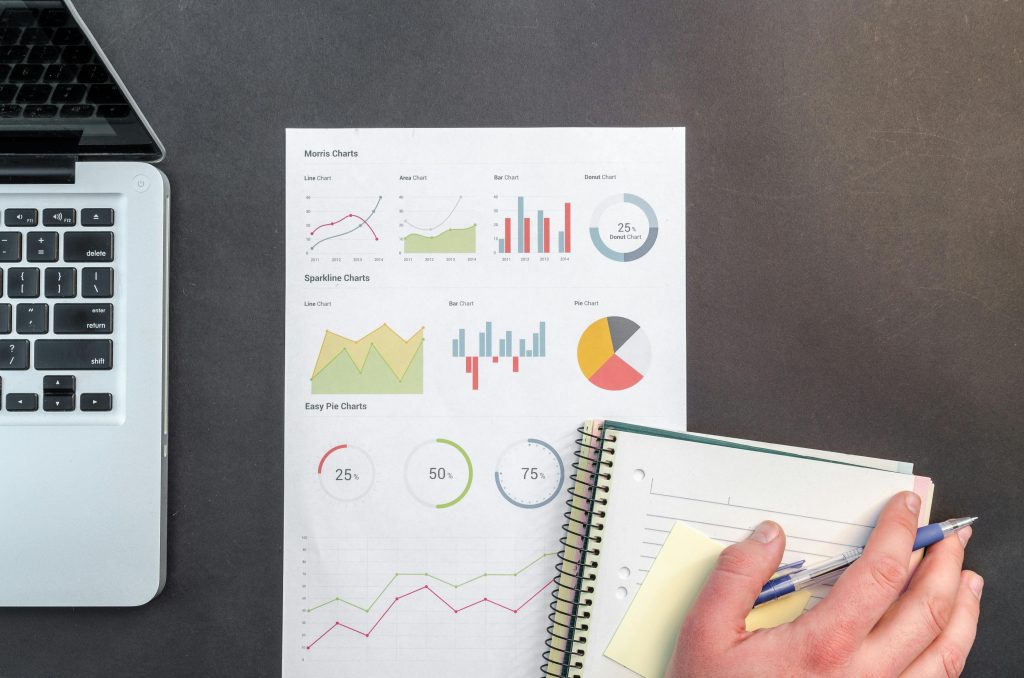
A data scientist is a professional who uses analytical expertise, programming skills, domain knowledge, and strong communication abilities to clean, process, and analyse data, build predictive models, and ethically communicate insights to drive informed decision-making. A data scientist has in-depth knowledge of mathematics, statistics and computer programming. He/she also possesses technical knowledge of Spreadsheet and Presentation tools .
The Intricacies of Data Science: Data Science Lifecycle
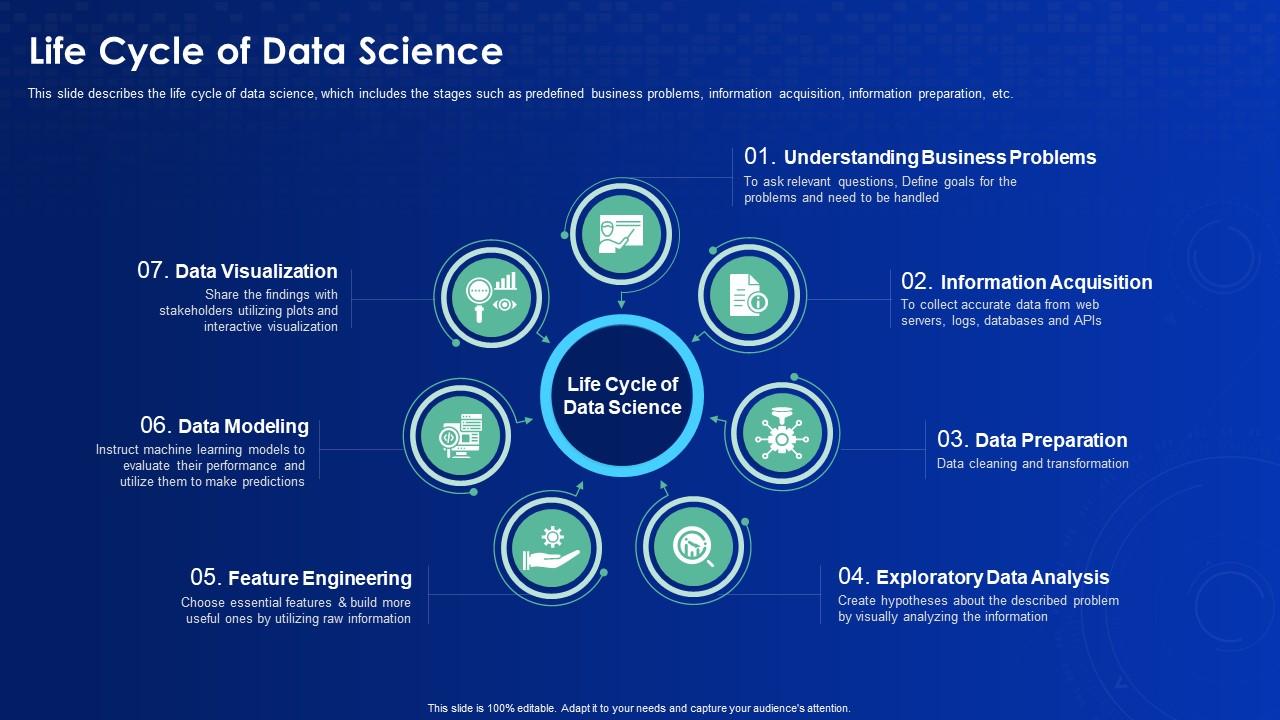
Having understood what the problem to be tackled is about, there are six phases in the Data Science Lifecycle:
1. Data Collection
This phase involves gathering raw data from various sources such as databases, APIs, web scraping, questionnaires and other data repositories.
2. Data Cleaning and Pre-processing
Data often comes with noise and inconsistencies. This phase focuses on handling missing values, removing duplicates, correcting errors, and transforming data into a suitable format for analysis. In fact, some of the data could be removed if found to be of negative or null impact to the model training.
3. Data Exploration and Analysis
Here, the data is thoroughly examined to discover patterns, correlations, and insights. Techniques such as statistical analysis, data visualisation, and exploratory data analysis (EDA) are used to understand the data better.
4. Model Building and Evaluation
In this phase, various statistical and mathematical algorithms are applied to the processed data to build models. These models are trained, validated, and tested to ensure they accurately predict or classify the target variable. Model performance is evaluated using metrics like accuracy, precision, recall, and F1-score.
5. Deployment and Monitoring
The final phase involves deploying the model into a production environment where it can be used to make real-time predictions. Continuous monitoring is essential to ensure the model’s performance remains consistent over time, and any necessary updates or retraining can be performed. This phase is majorly left to the Machine Learning Engineer (MLE).
6. Data Storytelling
This phase is arguably the most important, as it focuses on clearly and compellingly communicating the insights and findings derived from the data. By using data visualization, narratives, and actionable insights, data storytelling helps stakeholders understand the implications and make informed decisions. Effective storytelling is crucial because the value of data science lies in its ability to inform and drive decisions. Data scientists often need to present their findings to individuals with limited knowledge of mathematics and statistics, making clear communication essential.
Applications of Data Science
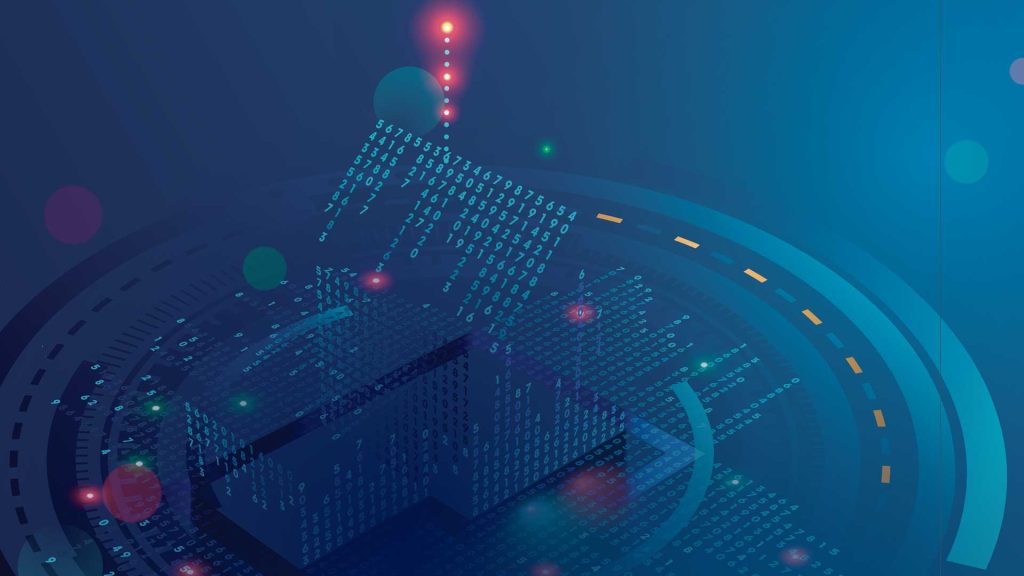
Data science is applied in various industries to solve complex problems and make important decisions:
- Healthcare: Predicting disease outbreaks, personalizing treatment plans, and optimizing hospital operations. The most example is cancer prediction using Image recognition.
- Finance: Detecting fraudulent transactions, credit scoring, and algorithmic trading. Machine learning algorithms are trained based on previous data to identify fraudulent transactions and also predict stock/forex/cryptocurrency prices. Models are also trained to project company sales, government budgets etc, based on this projections, businesses and governments can make decisions or policies to cope with impending crisis or further boost the economy.
- Retail: Inventory management, customer segmentation, and personalized marketing.
- Transportation: Route optimization, demand forecasting, and autonomous vehicles. Google maps and other services like it are products of data science, agents are built on data to navigate roads.
- Social Media: Sentiment analysis, recommendation systems, and content moderation, these are all products of data science.
- Weather Forecasting: Weather forecasting services operate on APIs whose major components are predictive models trained on climate data collected over several years.
Conclusion
Data science is transforming the way organizations operate by providing deeper insights and enabling data-driven decision-making. As data continues to grow exponentially, the demand for skilled data scientists is also on the rise. Whether it’s predicting customer behavior, optimizing business processes, or uncovering new opportunities, understanding the intricacies of data science is crucial in today’s data-centric world.
Phytonista🫡
🔥
Thank you for the insights